Statistics using SimpleITK#
We can use SimpleITK for extracting features from label images. For convenience reasons we use the napari-simpleitk-image-processing library.
import numpy as np
import pandas as pd
from skimage.io import imread
from pyclesperanto_prototype import imshow
from skimage import measure
import pyclesperanto_prototype as cle
from skimage import filters
from napari_simpleitk_image_processing import label_statistics
# load image
image = imread("../../data/blobs.tif")
# denoising
blurred_image = filters.gaussian(image, sigma=1)
# binarization
threshold = filters.threshold_otsu(blurred_image)
thresholded_image = blurred_image >= threshold
# labeling
label_image = measure.label(thresholded_image)
# visualization
imshow(label_image, labels=True)
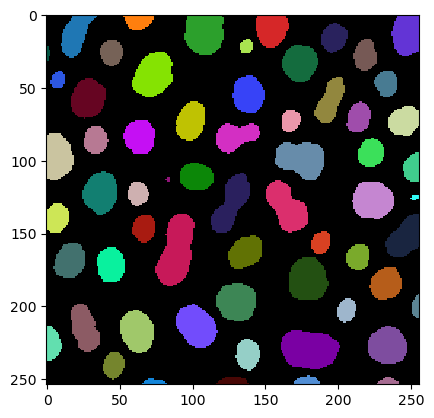
Measurements/ region properties#
We are now using the very handy function label_statistics
which provides a table of features. Let us check first what we need to provide for this function:
label_statistics?
Signature:
label_statistics(
intensity_image: 'napari.types.ImageData',
label_image: 'napari.types.LabelsData',
size: bool = True,
intensity: bool = True,
perimeter: bool = False,
shape: bool = False,
position: bool = False,
moments: bool = False,
napari_viewer: 'napari.Viewer' = None,
) -> 'pandas.DataFrame'
Docstring:
Measure intensity/shape/... statistics per label
Parameters
----------
intensity_image: ndarray, optional
Can be None
label_image: ndarray
Must be subsequently labeled
size: bool, optional
intensity: bool, optional
perimeter: bool, optional
shape: bool, optional
position: bool, optional
moments: bool, optional
napari_viewer: napari.Viewer, optional
Returns
-------
pandas DataFrame, in case napari_viewr is None, otherwise the DataFrame will be added to
the passed label_image's layer.features
See Also
--------
..[0] https://simpleitk.org/doxygen/latest/html/classitk_1_1simple_1_1LabelShapeStatisticsImageFilter
..[1] http://insightsoftwareconsortium.github.io/SimpleITK-Notebooks/Python_html/35_Segmentation_Shape_Analysis.html
File: c:\users\maral\mambaforge\envs\feature_blogpost\lib\site-packages\napari_simpleitk_image_processing\_simpleitk_image_processing.py
Type: function
Feature categories which have are set to True
are measured by default. In this case, the categories are size
and intensity
. But the rest might be also interesting to investigate. So we need them to True
as well:
df = pd.DataFrame(label_statistics(image, label_image,
shape=True,
perimeter=True,
position=True,
moments=True))
df
label | maximum | mean | median | minimum | sigma | sum | variance | bbox_0 | bbox_1 | ... | number_of_pixels_on_border | perimeter | perimeter_on_border | perimeter_on_border_ratio | principal_axes0 | principal_axes1 | principal_axes2 | principal_axes3 | principal_moments0 | principal_moments1 | |
---|---|---|---|---|---|---|---|---|---|---|---|---|---|---|---|---|---|---|---|---|---|
0 | 1 | 232.0 | 191.440559 | 200.0 | 128.0 | 29.827923 | 82128.0 | 889.704987 | 10 | 0 | ... | 16 | 87.070368 | 16.0 | 0.183759 | 0.905569 | 0.424199 | -0.424199 | 0.905569 | 17.336255 | 75.599678 |
1 | 2 | 224.0 | 179.846995 | 184.0 | 128.0 | 21.328889 | 32912.0 | 454.921516 | 53 | 0 | ... | 21 | 53.456120 | 21.0 | 0.392846 | -0.042759 | -0.999085 | 0.999085 | -0.042759 | 8.637199 | 27.432794 |
2 | 3 | 248.0 | 205.604863 | 208.0 | 120.0 | 29.414615 | 135288.0 | 865.219581 | 95 | 0 | ... | 23 | 93.409370 | 23.0 | 0.246228 | 0.989650 | 0.143505 | -0.143505 | 0.989650 | 49.994764 | 56.996778 |
3 | 4 | 248.0 | 217.515012 | 232.0 | 120.0 | 35.893817 | 94184.0 | 1288.366094 | 144 | 0 | ... | 20 | 76.114262 | 20.0 | 0.262763 | 0.902854 | 0.429947 | -0.429947 | 0.902854 | 33.290649 | 37.542552 |
4 | 5 | 248.0 | 213.033898 | 224.0 | 128.0 | 28.771575 | 100552.0 | 827.803519 | 237 | 0 | ... | 39 | 82.127941 | 40.0 | 0.487045 | 0.999090 | 0.042642 | -0.042642 | 0.999090 | 24.209327 | 60.391416 |
... | ... | ... | ... | ... | ... | ... | ... | ... | ... | ... | ... | ... | ... | ... | ... | ... | ... | ... | ... | ... | ... |
57 | 58 | 224.0 | 184.525822 | 192.0 | 120.0 | 28.322029 | 39304.0 | 802.137302 | 39 | 232 | ... | 0 | 52.250114 | 0.0 | 0.000000 | 0.976281 | 0.216509 | -0.216509 | 0.976281 | 13.084485 | 21.981750 |
58 | 59 | 248.0 | 184.810127 | 184.0 | 128.0 | 33.955505 | 14600.0 | 1152.976306 | 170 | 248 | ... | 18 | 39.953250 | 18.0 | 0.450527 | -0.012197 | -0.999926 | 0.999926 | -0.012197 | 2.075392 | 20.902016 |
59 | 60 | 216.0 | 182.727273 | 184.0 | 128.0 | 24.557101 | 16080.0 | 603.051202 | 117 | 249 | ... | 22 | 46.196967 | 22.0 | 0.476222 | -0.014920 | -0.999889 | 0.999889 | -0.014920 | 1.815666 | 29.359308 |
60 | 61 | 248.0 | 189.538462 | 192.0 | 128.0 | 38.236858 | 9856.0 | 1462.057315 | 227 | 249 | ... | 15 | 32.924135 | 15.0 | 0.455593 | -0.013675 | -0.999906 | 0.999906 | -0.013675 | 1.592570 | 12.843450 |
61 | 62 | 224.0 | 173.833333 | 176.0 | 128.0 | 28.283770 | 8344.0 | 799.971631 | 66 | 250 | ... | 17 | 35.375614 | 17.0 | 0.480557 | 0.030675 | -0.999529 | 0.999529 | 0.030675 | 0.917610 | 17.904873 |
62 rows × 33 columns
These are all columns that are available:
print(df.keys())
Index(['label', 'maximum', 'mean', 'median', 'minimum', 'sigma', 'sum',
'variance', 'bbox_0', 'bbox_1', 'bbox_2', 'bbox_3', 'centroid_0',
'centroid_1', 'elongation', 'feret_diameter', 'flatness', 'roundness',
'equivalent_ellipsoid_diameter_0', 'equivalent_ellipsoid_diameter_1',
'equivalent_spherical_perimeter', 'equivalent_spherical_radius',
'number_of_pixels', 'number_of_pixels_on_border', 'perimeter',
'perimeter_on_border', 'perimeter_on_border_ratio', 'principal_axes0',
'principal_axes1', 'principal_axes2', 'principal_axes3',
'principal_moments0', 'principal_moments1'],
dtype='object')
df.describe()
label | maximum | mean | median | minimum | sigma | sum | variance | bbox_0 | bbox_1 | ... | number_of_pixels_on_border | perimeter | perimeter_on_border | perimeter_on_border_ratio | principal_axes0 | principal_axes1 | principal_axes2 | principal_axes3 | principal_moments0 | principal_moments1 | |
---|---|---|---|---|---|---|---|---|---|---|---|---|---|---|---|---|---|---|---|---|---|
count | 62.000000 | 62.000000 | 62.000000 | 62.000000 | 62.000000 | 62.000000 | 62.000000 | 62.000000 | 62.000000 | 62.000000 | ... | 62.000000 | 62.000000 | 62.000000 | 62.000000 | 62.000000 | 62.000000 | 62.000000 | 62.000000 | 62.000000 | 62.000000 |
mean | 31.500000 | 233.548387 | 190.429888 | 196.258065 | 125.161290 | 28.767558 | 69534.451613 | 864.079980 | 121.532258 | 112.629032 | ... | 5.564516 | 67.071263 | 5.580645 | 0.102864 | 0.703539 | -0.114112 | 0.114112 | 0.703539 | 20.742486 | 43.535735 |
std | 18.041619 | 19.371838 | 15.382559 | 19.527144 | 4.602898 | 6.091478 | 42911.182492 | 311.860596 | 80.574925 | 76.921129 | ... | 9.667625 | 23.507575 | 9.724986 | 0.173858 | 0.459158 | 0.537821 | 0.537821 | 0.459158 | 13.092549 | 32.666912 |
min | 1.000000 | 152.000000 | 146.285714 | 144.000000 | 112.000000 | 6.047432 | 1024.000000 | 36.571429 | 0.000000 | 0.000000 | ... | 0.000000 | 9.155272 | 0.000000 | 0.000000 | -0.651619 | -0.999926 | -0.660963 | -0.651619 | 0.489796 | 0.571429 |
25% | 16.250000 | 232.000000 | 182.969505 | 186.000000 | 120.000000 | 26.561523 | 36010.000000 | 705.524752 | 53.000000 | 43.250000 | ... | 0.000000 | 52.551616 | 0.000000 | 0.000000 | 0.752633 | -0.624188 | -0.318631 | 0.752633 | 11.068721 | 22.027192 |
50% | 31.500000 | 240.000000 | 190.749492 | 200.000000 | 128.000000 | 29.089645 | 71148.000000 | 846.217207 | 121.000000 | 110.500000 | ... | 0.000000 | 68.204464 | 0.000000 | 0.000000 | 0.937869 | 0.040942 | -0.040942 | 0.937869 | 18.744728 | 35.367902 |
75% | 46.750000 | 248.000000 | 199.725305 | 208.000000 | 128.000000 | 32.583571 | 99962.000000 | 1061.691674 | 197.250000 | 172.250000 | ... | 12.500000 | 84.307520 | 12.500000 | 0.184963 | 0.994807 | 0.318631 | 0.624188 | 0.994807 | 29.519182 | 56.938641 |
max | 62.000000 | 248.000000 | 220.026144 | 240.000000 | 136.000000 | 38.374793 | 177944.000000 | 1472.624704 | 251.000000 | 250.000000 | ... | 39.000000 | 125.912897 | 40.000000 | 0.487045 | 1.000000 | 0.660963 | 0.999926 | 1.000000 | 49.994764 | 186.225041 |
8 rows × 33 columns
Specific measures#
SimpleITK offers some non-standard measurements which deserve additional documentation
number_of_pixels_on_border
#
First, we check its range on the above example image.
number_of_pixels_on_border = df['number_of_pixels_on_border'].tolist()
np.min(number_of_pixels_on_border), np.max(number_of_pixels_on_border)
(0, 39)
Next, we visualize the measurement in space.
number_of_pixels_on_border_map = cle.replace_intensities(label_image, [0] + number_of_pixels_on_border)
number_of_pixels_on_border_map
|
cle._ image
|
The visualization suggests that number_of_pixels_on_border
is the count of pixels within a label that is located at the image border.
perimeter_on_border
#
perimeter_on_border = df['perimeter_on_border'].tolist()
np.min(perimeter_on_border), np.max(perimeter_on_border)
perimeter_on_border_map = cle.replace_intensities(label_image, [0] + perimeter_on_border)
perimeter_on_border_map
|
cle._ image
|
The visualization suggests that perimeter_on_border
is the perimeter of labels that are located at the image border.
perimeter_on_border_ratio
#
In this context, the SimpleITK documentation points to a publication which mentiones “‘SizeOnBorder’ is the number of pixels in the objects which are on the border of the image.” While the documentation is wage, the perimeter_on_border_ratio
may be related.
perimeter_on_border_ratio = df['perimeter_on_border_ratio'].tolist()
np.min(perimeter_on_border_ratio), np.max(perimeter_on_border_ratio)
perimeter_on_border_ratio_map = cle.replace_intensities(label_image, [0] + perimeter_on_border_ratio)
perimeter_on_border_ratio_map
|
cle._ image
|
The visualization reinforces our assumption that the perimeter_on_border_ratio
indeed is related to the amount the object touches the image border.
principal axes
#
In our example, we have principal_axes0
- principal_axes3
principal_axes_0 = df['principal_axes0'].tolist()
np.min(principal_axes_0), np.max(principal_axes_0)
principal_axes_0_map = cle.replace_intensities(label_image, [0] + principal_axes_0)
principal_axes_0_map
|
cle._ image
|
(principal_axes3
looks the same)
principal_axes_1 = df['principal_axes1'].tolist()
np.min(principal_axes_1), np.max(principal_axes_1)
principal_axes_1_map = cle.replace_intensities(label_image, [0] + principal_axes_1)
principal_axes_1_map
|
cle._ image
|
principal_axes_2 = df['principal_axes2'].tolist()
np.min(principal_axes_2), np.max(principal_axes_2)
principal_axes_2_map = cle.replace_intensities(label_image, [0] + principal_axes_2)
principal_axes_2_map
|
cle._ image
|
principal moments
#
principal_moments_0 = df['principal_moments0'].tolist()
np.min(principal_moments_0), np.max(principal_moments_0)
principal_moments_0_map = cle.replace_intensities(label_image, [0] + principal_moments_0)
principal_moments_0_map
|
cle._ image
|
principal_moments_1 = df['principal_moments1'].tolist()
np.min(principal_moments_1), np.max(principal_moments_1)
principal_moments_1_map = cle.replace_intensities(label_image, [0] + principal_moments_1)
principal_moments_1_map
|
cle._ image
|