Select labels using machine learning#
apoc allows selecting labeled objects according to properties such as size, shape and intensity in a corresponding image. In this example, we will select elongated objects from an instance segmentation of blobs.
import apoc
from skimage.io import imread
import pyclesperanto_prototype as cle
import numpy as np
cle.select_device('RTX')
<NVIDIA GeForce RTX 3050 Ti Laptop GPU on Platform: NVIDIA CUDA (1 refs)>
image = imread('../../data/blobs.tif')
labels = cle.label(cle.threshold_otsu(image))
annotation = imread('../../data/label_annotation.tif')
cle.imshow(image)
cle.imshow(labels, labels=True)
cle.imshow(annotation, labels=True)
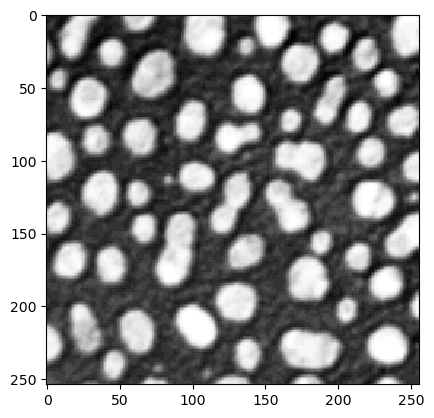
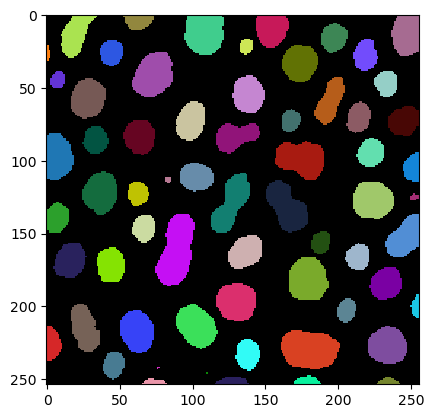
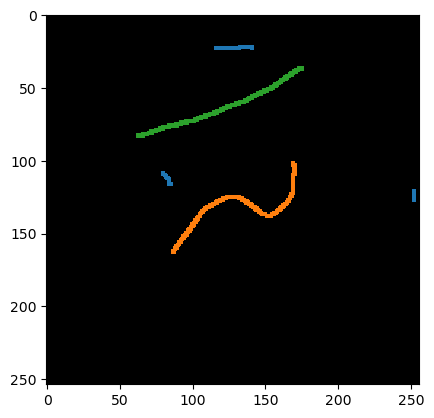
Training#
For training the classifier, you need to specify features. In the following we use mean and standard deviation intensity within the labeled objects and the object size and shape.
features = 'area,mean_max_distance_to_centroid_ratio,standard_deviation_intensity'
cl_filename = "object_selector.cl"
# Create an object classifier
apoc.erase_classifier(cl_filename) # delete it if it was existing before
classifier = apoc.ObjectSelector(cl_filename, positive_class_identifier=1)
# train it
classifier.train(features, labels, annotation, image)
Prediction#
After the classifier was trained, we can use it to select objects.
result = classifier.predict(labels, image)
print(result.max())
cle.imshow(result, labels=True)
23.0
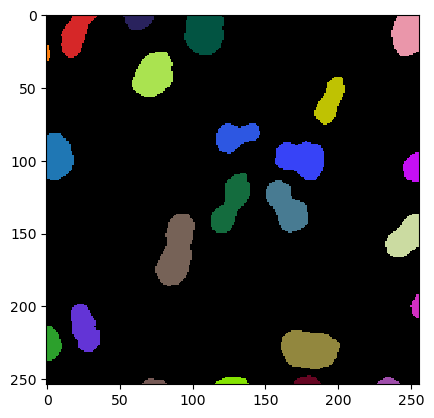
One can also load the classifier from disc and apply it to another dataset. We demonstrate that by applying the classifier to a rotated version of the image and label image from above.
image1 = image.T
labels1 = cle.label(cle.threshold_otsu(image1))
classifier = apoc.ObjectSelector(cl_filename)
result = classifier.predict(labels1, image1)
print(result.max())
cle.imshow(result, labels=True)
23.0
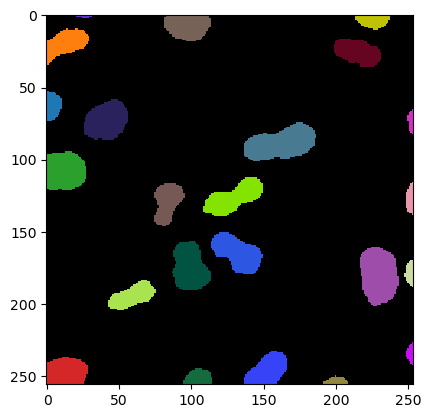
After training, we can ask the classifier how important features were while doing the prediction.
classifier.feature_importances()
{'area': 0.29573084473661354,
'mean_max_distance_to_centroid_ratio': 0.4264564597125618,
'standard_deviation_intensity': 0.27781269555082466}
Exercise#
Use the code and example images above to train a classifier that selects all small objects in the label image.